Delfina: Isabel Fulcher, VP Data Science
"What excites me the most is the opportunity to implement something totally new."
Science fiction. Many working in biotech or medicine argue that we are now living it.
They have a point. The past decade has seen the development of engineered immune cells that can seek and destroy tumors, viral delivery of CRISPR constructs to lower cholesterol or fix blood disorders, flexible RNA-based vaccines and advanced machine learning algorithms to detect cancer, predict protein structures or even generate novel drugs—to name just a few of the discoveries at the frontiers of science and medicine.
But what about the oldest branch of medicine? What tools do we have to keep mothers and their babies healthy? Why do some pregnant women develop dangerously high blood pressure or diabetes during pregnancy? How do subtle shifts in fetal heart rate predict outcomes, and what should we do about it? Why does the US have the highest maternal mortality rate among high-income countries, and how can future solutions be applied fairly to different populations?
Delfina, a San Francisco-based digital health startup, was founded to tackle these questions. Senan Ebrahim, MD-PhD (CEO) decided to start Delfina after his OBGYN rotation in medical school: “we had a stillbirth in one of our pregnant patients - a tragic loss which motivated me to study how we could predict preventable complications of pregnancy. I became obsessed,” Dr. Ebrahim tells Biomarker. Joined by co-founders Dr. Ali Ebrahim and Priyanka Vaidya, Ebrahim set out in 2020 to design a data platform to monitor, predict and help manage pregnancy risks. Drawing on the team’s deep expertise in machine learning, OBGYN and software development, Delfina uses bespoke datasets and proprietary algorithms to make tools for both patients and providers (Q #6-7). In 2021, the company participated in the NICHD Decoding Maternal Morbidity Challenge, where it received an innovation award for analyzing health disparities using its Hypertensive Disorders of Pregnancy Predictor. Their platform has recently made it into patient and provider hands: “We just launched Delfina Care with A&A Women's Health, an innovative OBGYN practice in Southern California. After this successful deployment, we are excited to scale our proactive care model widely to serve diverse populations” says Dr. Ebrahim. Delfina was also recently accepted into the Mayo Clinic’s Platform Accelerate cohort, where they will have access to large scale datasets to further train and improve their predictive models.
In March 2022, Delfina raised $5.3M in seed funding led by Story Ventures. Other participants in the round include SemperVirens VC, Bread & Butter Ventures, Goodwater Capital, MIT E14 Fund, Metrodora VC, SpringTime Ventures as well as angel investors including former CEO of Reddit Ellen Pao and former Facebook Head of VR Hugo Barra.
Dr. Isabel Fulcher is the VP of Data Science at Delfina. She holds a PhD in Biostatistics from Harvard and was previously a Harvard Data Science Initiative Postdoctoral Fellow. She is an expert in the field of causal inference, and her academic work used novel statistical methods to evaluate how interventional programs and policies impact maternal health outcomes. She has worked closely with research partners at Planned Parenthood, Partners In Health, D-tree International, and Mass General Brigham. Passionate about using her statistical chops and deep expertise in wrangling complex datasets to improve maternal health outcomes, she is a rising star in the FemTech space.
Below is an interview with Dr. Isabel Fulcher, VP Data Science at Delfina from September 2022:
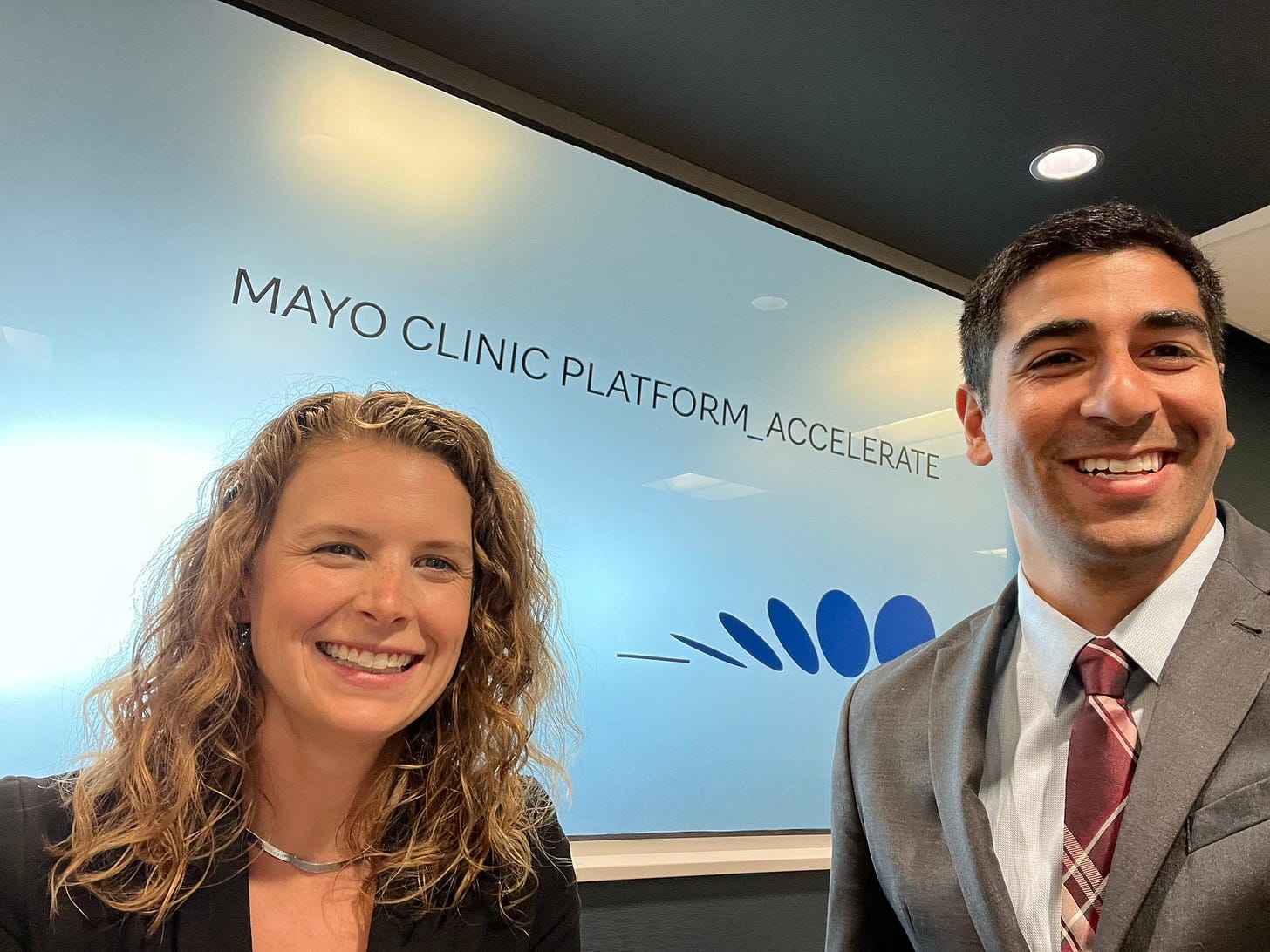
1. How did you first become interested in statistics and data science? Did you always envision applying these skills to human health?
I have been interested in math ever since I was young. When I attended McGill University, I was in the Arts and Science program, meaning that I had to choose both a science major and an arts major. Math was a given for me, and I landed on anthropology for my arts major. Although I was very interested in both subject areas, I pursued them independently as I had not yet found a way to naturally connect them. I distinctly remember a medical anthropology course piquing my interest in health. Reading the works of Arthur Kleinman, Paul Farmer and Nancy Scheper-Hughes changed how I thought about healthcare in the US and abroad. From there, I became very interested in human health and how humans engage within medical systems.
Prior to starting graduate school, I worked at a healthcare consulting company for two years. Although I was learning a ton, I had a desire to deeply understand statistics and be able to apply it to healthcare problems, which is what drove me to go back to get my PhD in biostatistics.
2. What is one really memorable experience from PhD that led you to your current work? How did you get involved in Delfina?
In graduate school, I worked with an organization called D-tree International, a nonprofit that uses digital tools to strengthen health systems. I spent the summer in Zanzibar teaching a hands-on data science course for their team and analyzing data collected through their maternal health program. The data science course focused on answering research questions specific to their maternal health program, while also teaching basic data science and epidemiological skills.
When analyzing data from the health program, we noticed that an alarmingly high proportion of pregnant women were delivering early. However, after chatting with community health workers, we realized that misestimation of gestational ages was probably the culprit. Gestational age was estimated based on the time of the last menstrual period, which was likely subject to errors for a variety of reasons. If we had taken the data at face value, we would have incorrectly concluded that the prevalence of preterm births was very high in Zanzibar.
This led to an ongoing collaboration with D-tree and research projects aimed at correcting gestational age measurements. Personally, the experience opened my eyes to how important it is to understand where your data is coming from and engaging with the individuals involved in the data collection process.
[On getting involved with Delfina]
I have been following the blossoming “FemTech” world over the past few years and was keeping a close eye on companies in the pregnancy care space. This past March, I saw that Delfina had raised a seed round and was building a data science team. Delfina’s mission really resonated with me based on my prior work in both digital health and reproductive health. As a researcher, I loved the emphasis on building digital technologies based on rigorous scientific evidence. I decided to reach out to the CTO Ali Ebrahim and the rest is history!
3. What is one book that has influenced the way you think, which you would recommend to folks interested in either science, medicine or stats?
I would recommend Killing the Black Body by Dorothy Roberts. In grad school, I started working with Planned Parenthood on a research study, and the book was recommended to me by the PI. The book completely reframed how I understood reproductive health as it details the violent history of the systematic abuse of black women's bodies in the United States. I think this should be required reading for anyone in the reproductive health space—especially those who are designing solutions to address the black maternal health crisis in the United States.
4. Briefly, what is causal inference? What is an example of how this is area of statistics is crucial to medicine?
Causal inference provides a framework for isolating the effect of an intervention or exposure on an outcome. To me, causal inference is necessary for rigorous medical research. It forces you to be very precise in defining both your causal question and the assumptions needed to answer that causal question.
In my research, I use data arising from electronic health records to investigate causal questions, which are often rife with missing data, measurement error, and may be missing important variables needed to control for “confounding”. Thankfully, the vast and ever-growing causal inference literature provides a suite of tools to account for these problems.
5. What is an interesting scientific paper you read recently that you would recommend to someone looking to learn more about causal inference and its applications to medicine? Leading labs in the field?
Here are two papers I came across recently that are excellent summaries of the current causal inference literature with health applications.
Primers papers:
1. Causal Inference Challenges and New Directions for Epidemiologic Research on the Health Effects of Social Policies, Matthay and Glymour, 2022
2. Reflection on modern methods: when worlds collide—prediction, machine learning and causal inference (Blakely et al., 2021).
There are so many people doing great work at the forefront of causal inference and public health! To get an understanding of the breadth of work, I would recommend the following researchers:
Maria Glymour, UCSF
Miguel Hernan, HMS
Edward Kennedy, Carnegie Mellon
Elizabeth Stuart, Johns Hopkins University
Eric Tchetgen Tchetgen, University of Pennsylvania
6. What excites you most about working at Delfina? Briefly, what is the magic sauce or competitive advantage?
What excites me the most is the opportunity to implement something totally new. Over the past five years, I have been involved in a lot of exciting research studies. Most of these studies investigated the barriers and disparities in reproductive health service utilization. Delfina feels like an opportunity to address those barriers by developing digital technologies that will improve pregnancy outcomes.
Our “magic sauce” is that we have all of the pieces in place to “get it right.” Delfina has assembled amazing clinical, engineering, data science, and product teams with deep, yet diverse expertise. For example, on the clinical side, we have clinicians from both academic medical institutions and community practices. Having this breadth of experience is key for us, because we are trying to solve the maternal health crisis across the US—a problem that does not just exist in major cities or academic medical centers. The ability to work with all these talented people is what really excites me, and I believe this is also our greatest strength.
7. Delfina has two platforms, one for families and one for providers. What types of data do you work with for each, and what are some of the challenges of working with complex and rich datasets?
We have a patient facing app that collects biometric data, such as blood pressure and weight, during pregnancy. The app not only enables the patient to visualize their own health trends, but also feeds back to a provider dashboard to support patient management and data-driven decision making. A challenge, inherent to any app, is getting patients to engage with the app, which involves solving issues of phone connectivity and technological literacy. This is an area where our product team’s expertise has really shone through by developing creative solutions for increasing patient engagement.
In tandem, we also work with large health datasets from across the United States to build prediction models for key pregnancy diagnoses and outcomes that are then embedded within the app. The problems that arise in electronic health record data include missing data and measurement error. Fortunately, our data science team has deep expertise in developing methods to deal with these issues as we build and deploy our models in a variety of contexts.
8. What are you currently working hardest on at Delfina on the data science side? What are the upcoming milestones you are using to keep your team motivated?
We were recently accepted into Mayo Clinic Platform Accelerate. Through this program, we have a lot of awesome workshops and resources to learn about Mayo Clinic’s electronic health record system. We also have access to the rich health data from the Mayo Clinic, and our data science team is working on building and validating models in this patient population. Basically, we are processing a lot of data and learning a ton!
9. For an investor looking at a healthcare IT/SaaS company using predictive modeling, what are some basic questions you should always ask?
First and foremost, how will the prediction model improve patient outcomes? Building a high performing prediction model only matters if it is actionable in a real-world setting. I would want to know how this specific model would change practice before diving deeper.
Then, I would want to know all the nitty gritty details that will help me assess model performance in various settings. Specifically, what is the data source and population underlying the development of this model? How does this data source and population reflect where it will be applied? Does the model’s performance change across key demographic groups? Has the model been validated in different patient populations?
Recently at the Mayo Clinic Platform Accelerate, we had an awesome talk from Google’s machine learning group, and they introduced model cards as a “nutrition label” for machine learning models. After determining the broad utility of the model, I think these model cards would cover basically any question you could ask about the model development, performance, and validation.
[Example model card from Google]
10. In an ideal world, if you could go back to grad school and take one more class from any Harvard department, what would this be?
Professor Susan Murphy teaches a statistical reinforcement learning class, which I believe is heavily influenced by her work with just in time adaptive interventions in the digital health space. She started teaching the class right after I finished my PhD, so I would probably go back for a front row seat!
11. What is another biotech (public or private) that you think serves as a paragon of an impactful company? What are the key learnings from this case study?
One company that has impressed me in the FemTech space is Tia. They are changing the delivery of female healthcare by integrating gynecology, primary health, and mental health in one space. I really admire how they have identified and addressed pain points in navigating care.
12. In the next 10-20 years how do you hope data science solutions (like Delfina) will transform patient experiences and care?
One of the major issues I have come to realize is that our medical system is a mess of siloed data systems. I believe siloed data systems are problematic for two reasons.
The first issue is that the current standard of care involves snapshot encounters between a patient and only one member of their care team. Important health data, like blood pressure measurements, are only captured during office visits, and may only be available to the clinician directly administering care. The Delfina Care platform leverages remote patient monitoring to more frequently collect important health data, while also improving information flow from the patient to their entire care team. I believe this coordination will improve pregnancy outcomes by enabling real-time data-driven decision making. I am also hopeful that patients will feel more supported during their pregnancy journey because of these digital solutions.
The second is that there is currently no central data system in the United States, which makes coordinating research efforts challenging. For issues like pre-eclampsia and gestational diabetes, there are still a ton of knowledge gaps, so research is imperative for improving patient outcomes. I am hopeful that our data coordination work will not only directly impact health outcomes, but also advance reproductive health research.